

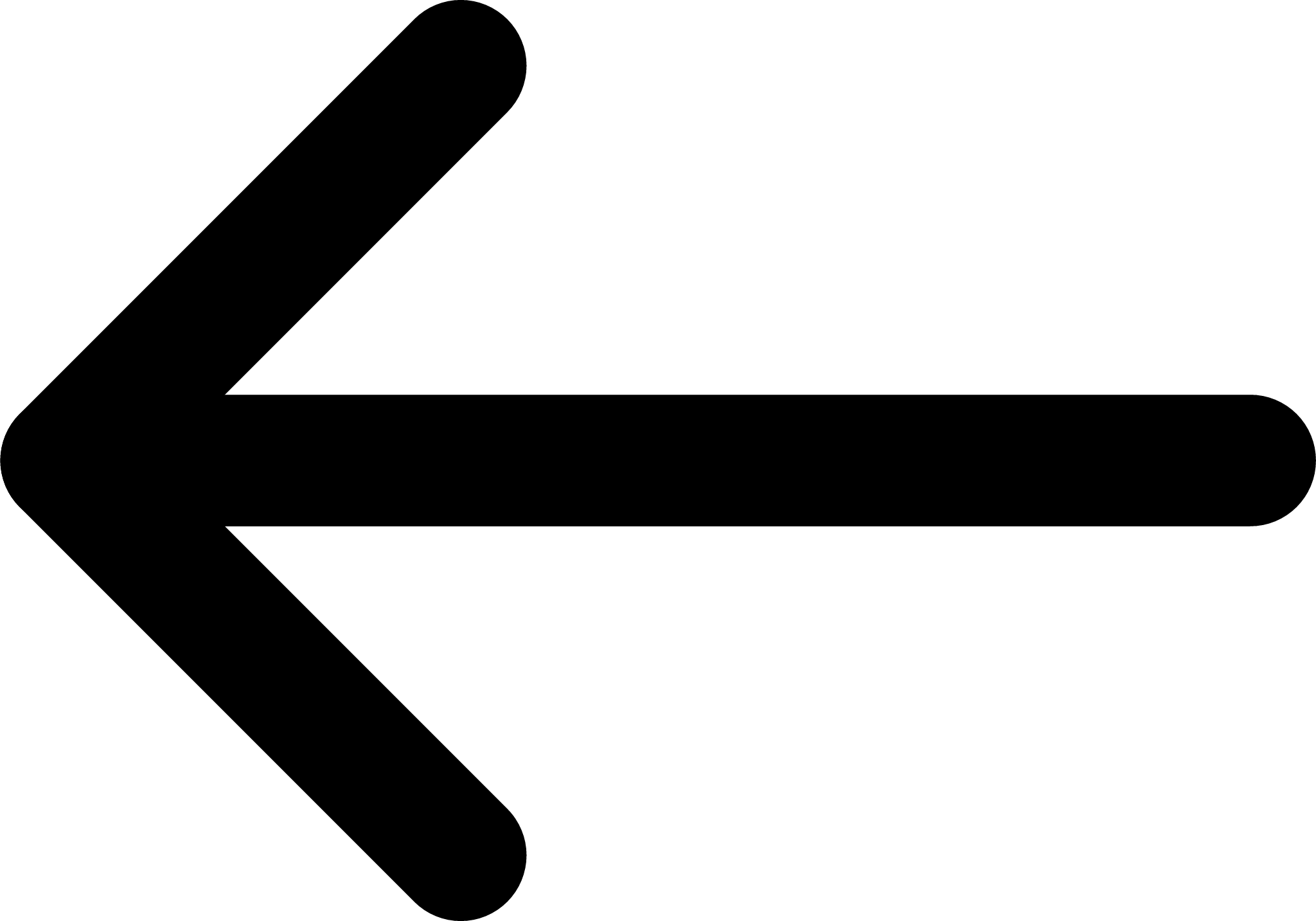
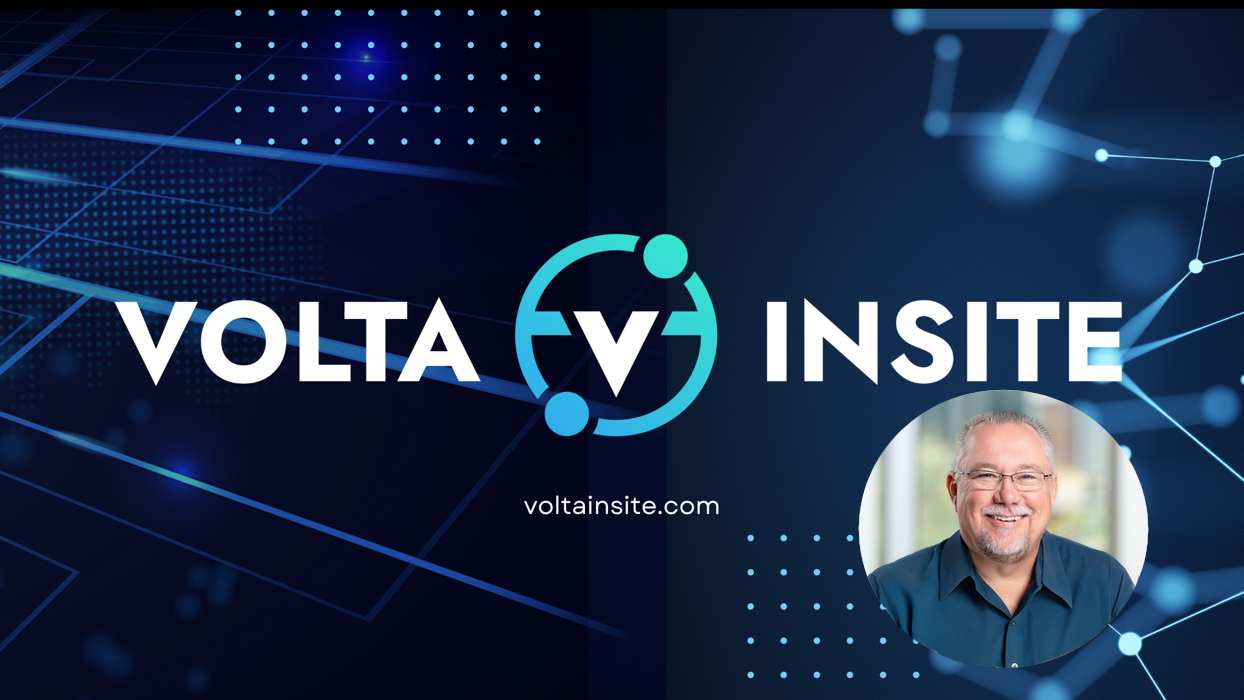
Predictive Maintenance & Monitoring in Power Systems
In North America and across the industrial world, unplanned downtime costs manufacturers tremendously, both in the immediate loss of production and in long-term damage to their machine tools and assets. To combat this, the maintenance strategy for critical equipment has evolved from a reactive posture—waiting for the machine to break down and then fixing it—to proactive maintenance measures. Proactive maintenance includes both Condition Monitoring and Predictive Maintenance. These approaches use advanced analyses and intelligent sensors to monitor machine health and predict potential failures before they result in costly downtimes.
Predictive Maintenance vs. Condition Monitoring
Condition monitoring serves as the industry's continuous health check-up. It records various parameters like vibration, temperature, and noise levels to monitor asset conditions in real time. The strategy relies on setting threshold levels that, when exceeded, trigger alerts. This prompts immediate inspection to determine the cause of the faulty operation.
On the other hand, Predictive Maintenance takes the strategy one step further. By aggregating sensor data and using insightful algorithms in a predictive maintenance solution, it not only detects anomalies but also analyzes patterns to forecast when a component or system might fail. This enables maintenance teams to schedule repairs or part replacements at an optimal time, thereby ensuring that operations remain uninterrupted.
Analyzing Differences and Complementarities
Condition Monitoring and Predictive Maintenance may seem similar, but they have distinct differences. The primary distinction lies in their application. Condition Monitoring puts the control firmly in the hands of the maintenance organization, with a key role played by sensor technologies and condition monitoring maintenance software sending real-time alerts when parameters indicate a deviation from normal operation.
Predictive Maintenance, alternatively, applies intelligent algorithms and Machine Learning (ML) to analyze trends over time. This allows for accurate predictions of when maintenance should occur, reducing the frequency of maintenance actions and preventing unnecessary costs associated with over-maintenance or untimely breakdowns.
Despite their differences, both strategies are complementary. Implementing condition monitoring solutions lays the groundwork for a solid predictive maintenance plan. Without the insights gained from Condition Monitoring, Predictive Maintenance would lack the detailed real-time data necessary for crafting accurate predictive algorithms.
Mutual Benefits of Integrating Both Strategies
When industries integrate Condition Monitoring and Predictive Maintenance, they harness a dual approach that maximizes machine uptime, enhances reliability, and minimizes downtime. The synergy between these strategies boosts maintenance effectiveness on multiple levels—Condition Monitoring enables immediate response to irregular conditions while Predictive Maintenance optimizes longer-term maintenance scheduling to avoid unforeseen machine failure.
Additionally, the incorporation of Artificial Intelligence (AI) and Predictive Analytics can refine these strategies. AI facilitates the extraction of subtle condition indicators from the vast amount of data that sensors collect, and predictive analytics visualize these findings to streamline decision-making processes.
By capitalizing on the mutual benefits and by utilizing tools like the Predictive Maintenance Toolbox, asset management software, and condition-based maintenance systems, maintenance leaders can ensure healthy operation. They are now positioned to predict, plan, and perform maintenance tasks in a way that saves valuable time and reduces overall maintenance expenses, ultimately protecting the bottom line against the high costs of unplanned downtime.
Core Concepts and Technologies Underpinning Predictive Maintenance
Leveraging Data: The Role of Preprocessing and Quality
In the pursuit of a reliable predictive maintenance program, the integrity of the data becomes non-negotiable. Preprocessing is the unsung hero here—it cleans, normalizes, and prepares data, sifting through each measurement to ensure what reaches the analysis stage represents a machine's genuine condition. This often involves filtering out noisy vibration signatures or isolating high-frequency sinusoidal components that may indicate early stages of gearbox anomalies. By maintaining a rigorous preprocessing protocol, predictive maintenance algorithms receive only the highest quality data, paving the way for precise fault diagnosis and accurate predictions.
Importance of Actionable Insights in Predictive Maintenance
An insight is only valuable if it leads to action. Predictive maintenance thrives on insights that maintenance teams can act upon—those that clearly indicate when to repair components or perform maintenance tasks like an oil change. Insights gained from analyzing data, trends, and changes over time are the difference between operation and downtime. They provide specific, actionable recommendations that save time for repairs before failure occurs.
The Use of Algorithms for Accurate Prognostics
Algorithms are the brains behind predictive maintenance, crafting future scenarios from current and historical data. They estimate the health and remaining useful life of an asset by comparing real-time performance against historical patterns, thresholds, and expected behaviors. Machine learning—especially its ability to adapt and learn from new data—plays a vital role in fine-tuning these predictive algorithms. With each machinery cycle, they grow smarter at picking out the subtlest hints of potential failure.
Advanced Data Analysis and Its Impact on Maintenance Decision-Making
Advanced data analysis transforms raw sensor data into a narrative about an asset’s health and future. Techniques such as vibration analysis, oil analysis, and thermal imaging, when combined with advanced analytics, provide a multi-dimensional view of equipment conditions. This rich, well-rounded understanding empowers maintenance managers to make informed decisions that optimize asset performance and predict when maintenance should occur with an impressive level of accuracy. The end result is a formidable reduction in unplanned downtime and costs, while upholding safety and reliability.
The study by Tawfik Borgi and colleagues presents a groundbreaking approach using data analytics for the predictive maintenance of industrial robots. Their research demonstrates the effectiveness of predictive modeling in identifying robot manipulator accuracy errors, further emphasizing the role of data-driven condition monitoring for enhancing operational efficiency and machine health.
Advances in Sensor and Data Collection Technologies for Monitoring
The Advent of Smart Sensor Nodes and Inertial Measurement Units
Smart sensor nodes are revolutionizing predictive maintenance by providing accurate, real-time monitoring of various machine parameters. Paired with Inertial Measurement Units (IMUs), these sensors can track and measure changes in vibration, rotational speed, and position to foresee potential issues with unparalleled precision. These intelligent sensors perform complicated analyses on the spot, preventing the need to send vast amounts of raw data over the network, thus saving on bandwidth and speeding up decision-making.
Edge Processing, Integrated Connectivity, and Communication Interfaces
Edge processing allows for immediate data preprocessing on machinery. This process reduces latency, making predictive maintenance more efficient by handling data close to its source. Integrated connectivity options, like wireless or Ethernet, enable these devices to communicate swiftly, while advanced communication interfaces ensure seamless integration into existing industrial systems. This ensures that the data not only flows fast but also accurately across all touchpoints of the predictive maintenance ecosystem.
The Expansion from Contact-Based to Contactless Torque Monitoring
Progressing beyond traditional methods, the rise of contactless torque sensors has set a new standard in monitoring the performance and condition of equipment. This innovative approach eliminates mechanical contact, meaning less wear and tear and lower maintenance costs. Thanks to their non-intrusive design, contactless sensors provide a precise and uninterrupted view of torque in real time, revealing insights into the health of assets that were previously hidden by the limitations of contact-based systems.
The Significance of Predictive Analytics in Condition Monitoring
Ensemble Data Processing and Condition Indicators Identification
Predictive analytics uses ensemble data processing to merge readings from various sensors into a single, more robust prediction model. This involves an ensemble variable approach, wherein multiple variables are assessed together to diagnose equipment health more accurately. Identifying condition indicators—the tell-tale signs that components may soon fail—is key. These indicators are like puzzle pieces that, when assembled correctly, provide a clear picture of the equipment's future needs.
Frequency-Domain and Time-Frequency Data Preprocessing Techniques
Preprocessing techniques transform raw data into a format that is easier for predictive maintenance algorithms to understand and analyze. Frequency-domain approaches look at how different vibration frequencies correlate with machine faults. Time-frequency techniques go a step further, analyzing how these frequencies change over time, which can reveal transient or evolving issues that might otherwise go unnoticed until it's too late.
Anomaly Detection and Artificial Intelligence Enhancements
Anomaly detection algorithms, bolstered by Artificial Intelligence (AI), spot deviations from normal operation, acting like a sophisticated tripwire for potential equipment problems. AI empowers these systems to learn what 'normal' looks like and then raise the alarm when something is out of the ordinary. This blend of condition monitoring and AI forms a powerful tool in the predictive maintenance arsenal, transforming data into insightful, actionable information that can drastically minimize downtime risks.
Overcoming the Challenges of Implementing Predictive Maintenance
Best Practices for Implementing Predictive Maintenance
To effectively implement predictive maintenance, it's essential to start with a solid foundation. Begin by establishing clear goals and understanding the specific needs of the equipment. Regularly train maintenance staff on the latest tools and encourage a culture that values proactive maintenance. Starting small and scaling as you see successes ensures manageable growth of predictive strategies. Continuous review and refining of processes, based on feedback and data analysis, keep the system performing at its best.
The Importance of Tailoring Strategies to Specific Machine Asset Types
Each machine asset comes with its own set of challenges and nuances. Tailoring predictive maintenance approaches to individual asset types is crucial for success. For example, a gearbox or other system component may require monitoring for specific frequency bands, while a fan might need a focus on vibration patterns. By customizing strategies to fit each machine's characteristics, factories can ensure they detect issues with greater accuracy, thereby saving resources and avoiding generic, less effective solutions.
Cost and ROI Calculation for Predictive Maintenance Initiatives
When considering the implementation of predictive maintenance, a thorough cost-benefit analysis is key. Measure the current cost of unplanned downtime, maintenance expenses, and parts against the projected cost of setting up and running a predictive maintenance system. Predictive maintenance should ultimately lead to a Reduction In Maintenance costs (RIM), and the calculation of Return on Investment (ROI) should capture not just immediate savings but also the increased longevity and performance of assets. Quantifying these benefits can present a clear picture of the financial advantage of predictive maintenance initiatives.
Real-World Applications of Predictive Maintenance
Predictive Maintenance in the Context of Different Industrial Assets
Predictive maintenance isn't a one-size-fits-all solution; it's remarkably versatile and tailored to various industrial assets. For instance, in electric motors, it predicts bearing failures or electrical issues, while in conveyor systems, it might anticipate belt misalignments or joint wear. In HVAC systems, predictive maintenance forecasts filter or refrigerant issues, ensuring comfortable and safe environments. By linking each asset's unique operational profile with the right predictive maintenance technologies, facilities can catch the early warning signs of failure across diverse applications.
Condition Monitoring in the Renewable Energy Sector
The renewable energy sector, particularly wind farms, leans heavily on condition monitoring to anticipate maintenance needs. Vibration analysis and oil debris monitoring are used to protect critical components like gearboxes and generators. Predictive maintenance ensures that turbines run optimally and helps to schedule maintenance during low-wind periods to minimize energy production losses. It's a game-changer for energy providers looking to maximize uptime, protect critical assets, and keep energy flowing without interruption.
Industrial Case Studies: Identifying Defects Before They Affect Production
Case studies across manufacturing, oil and gas, and automotive industries showcase predictive maintenance's pivotal role in preempting breakdowns. For example, a car manufacturer might use predictive maintenance to prevent unexpected downtimes of robotic assembly lines, while a petroleum refinery could employ it to avoid costly failures in high-pressure systems. These real-world success stories underscore the value of predictive maintenance in spotting defects that could pause production, proving its role as a cornerstone of modern industrial reliability and efficiency.
Key Takeaways
-
Cost Savings: Predictive maintenance cuts costs by reducing emergency repairs, preventing extensive downtime, and prolonging equipment life.
-
Eco-Friendly: This approach supports a sustainable manufacturing ecosystem through energy savings and waste reduction.
-
Compatibility: Modern predictive maintenance systems are designed to integrate seamlessly with existing PLC architectures, enhancing their capabilities for smart monitoring.
FAQs
What Are the Main Cost Benefits of Switching to Predictive Maintenance?
Switching to predictive maintenance brings significant cost benefits including reduced downtime, extended equipment life, fewer repairs, and better use of maintenance resources. By catching problems early, factories spend less time repairing and more time producing. Predictive maintenance also means fewer emergency calls for mechanics and electricians, which can be pretty expensive. In the long run, you'll spend less money on parts and labor, because maintaining equipment regularly keeps it running better and longer.
How Does Predictive Maintenance Contribute to Sustainability in Manufacturing?
Predictive maintenance has a green side, too. It helps make manufacturing more sustainable by cutting down waste. When machines run smoothly, they use less energy. There's also less need for spare parts, which means fewer resources used. Predictive maintenance helps catch leaks and emissions before they get worse, so it's also good for keeping the air and water clean. By extending the life of equipment, it reduces the need to produce or dispose of machinery, lowering the manufacturing footprint on the environment.
Can Predictive Maintenance Systems Be Integrated With Existing PLC Architectures?
Yes, predictive maintenance systems can definitely fit in with existing Programmable Logic Controller (PLC) setups. It's like giving your PLCs a new set of superpowers. They can now collect and send data to the predictive maintenance software, which will analyze all the info and let you know if something's starting to go wrong. This integration makes it possible to keep an eye on the health of your machines without having to start over with new equipment.